Accelerated by increasing digitalization and connectivity in manufacturing, the volume, velocity and variety of manufacturing data are growing exponentially. Manufacturers are seeking possibilities to leverage these massive datasets in order to acquire and apply new knowledge and skills, or “advanced manufacturing intelligence”, within their production environments. As a result, manufacturing analytics has become a conceptually and technically demanding field tying together big data, distributed computing, statistics and machine learning in order to produce valuable insights and detect trends, patterns, and anomalies in production data. Clearly, the goal is timely and actionable data – the question is: How?
To begin, identify a specific objective or question. For instance, if product quality improvements in semiconductor production are the objective, tool state and product quality measurements must be integrated over a period of time. Then, identify the data sources of interest. Perhaps sensor readings from the shop floor should be considered as dust and temperature fluctuations could be negatively affecting product quality. Another potentially relevant factor could be queue times at individual processing steps which may be affecting product quality and might need to be integrated. Once the objective is clear and data sources of interest have been identified, it is possible to begin the careful process of integrating these data streams. From there, machine learning, model selection, evaluation and operationalization can get underway. The resulting data analysis can quickly reveal root causes of issues affecting quality, yield or any number of other key performance measurements.
Meticulous Data Integration: A key factor for success with advanced manufacturing analytics
Data integration alone can be a challenging task. The selection and proper enrichment of relevant data is, in many cases, not just a technical problem but requires a detailed and in-depth knowledge of physio-chemical, electrical or mechanical properties of the manufacturing steps to be optimized. Adding to this complexity, data sets hardly ever fit together right away so a rigorous analytical approach (along with the development of data integrity test protocols when combining these data) is critical to provide meaningful insights. The good news? Collaboration among process engineers, data engineers and scientists make it possible to solve this challenging problem and lay the foundation for many applications to streamline production.
Figure 1. Data from various sources commonly accumulates and becomes siloed, making it difficult to aggregate and analyze.
Often, critical data sources aren’t available due to lack of equipment integration, absence of condition monitoring, incomplete product quality monitoring, or gaps in material tracking. Rectifying these gaps in connectivity enables data collection and provides rich and reliable sources for analysis and reporting that drive continuous improvements, optimizations, and efficiencies throughout the organization.
Business Intelligence Tools
Manufacturing enterprises commonly look to off-the-shelf business intelligence (BI) solutions to gain data insights. While BI solutions can be very powerful tools for enterprises dealing with low-volume, structured data sets, they fall short when it comes to the complexities associated with most high-tech manufacturing environments.
Highly automated factories easily produce terabytes of data per day. Compounding the complexities associated with extremely high amounts and varieties of data, every tool and process tends to be a unicorn, data structures and formats are highly diverse and dissimilar. Further, BI solutions are often not designed for the more complex technical, physical and chemical data characteristics of manufacturing processes that need to be monitored and optimized in today’s Industry 4.0 facilities. These situations call for more sophisticated solutions as high volumes of data need to be monitored and aggregated in real-time.
Use Cases
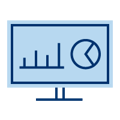
WIP Management
A common challenge in discrete manufacturing are material accumulations that slowly propagate through the factory. This can be addressed in multiple ways using manufacturing analytics. First, these accumulations need to be detected and tracked to identify the underlying root causes. Second, by adjusting scheduling and material influx, these bottlenecks can potentially be avoided. Finally, by forecasting material load (WIP) on tool level, bottlenecks can be anticipated and resolved before they occur.
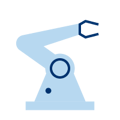
OEE Monitoring
Machines only make money if they are in use. Identifying and monitoring machine usage by metrics such as Overall Equipment Effectiveness (OEE) helps to reduce unscheduled tool down time and reveals efficiency gaps in production. Often, more advanced techniques are required which take into account not just tools but also operators, WIP and processes to enable valuable insights on root causes of ineffeciencies. By implementing an integrative holistic view on human, machine, material and methods it becomes possible to identify root causes at any level. This approach defines a clear analytical process to indicate efficiency and resource bottlenecks to cut down cycle times and to thus raise profitability.
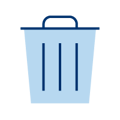
Waste Reduction
Material loss, or scrap, is costly and must be avoided where possible. There are a multitude of factors that can affect scrap rate. Carefully planned and implemented systems integrations ensure efficient identification and resolution of underlying root causes. Monitoring product quality with the aid of sensors, along with active communication among cooperating equipment and systems, expedites root cause identification related to equipment wear, environmental factors, and/or inefficient tool usage.
What We Offer
Provide Visibility into Factory Processes
Commonly, manufacturers have a wide variety of data sources, multiple production sites, and disparate systems running alongside specific questions that could be answered through careful analysis of their data. Unfortunately, most lack the ability to bring these data together for analysis. SYSTEMA’s approach involves the collection and aggregation of previously siloed data from sources such as FDC, equipment logs, message buses, material tracking systems, SPC, sensors, MES systems and more, in a way that mathematical and statistical analysis becomes possible to enable context enrichment. The results can provide admins, process owners, and management with a rich view of what is actually happening across their manufacturing sites.
Figure 2. By aggregating and enriching data in real-time, it is possible to gain insights into the current state of production. Long-term storage of this data is then warehoused to enable further analytical capabilities such as forecasting, process optimization and root cause analysis.
Remove Delays Between Operations and Reporting
Minimizing data latency and friction ensures that actionable intelligence is delivered within seconds to operator panels, control centers and management through various channels such dashboards and notifications.
The key to successful real-time factory monitoring is not merely the presentation of the current state, but to relate the current state to the historical context. Historical data analysis provides context and reveals deviations such as unexpected process time, uncommon material accumulations, or issues with material transport.
Enable Automatic, Data-Driven Decision Making
Machine learning enables the capture and modeling of inherent but hidden factory dynamics and enables solutions such as predictive maintenance, root cause analysis and load forecasting. Using deep learning methodologies, data-driven insights can be delivered that are central to the optimization of your unique factory processes. There are many examples where artificial intelligence (AI) has superseded human capabilities in scheduling, material routing, and process control.
Find the True Root Cause of Product Quality Issues
There are a huge number of variables that can affect product quality on various levels. In the case of sudden changes or high variability in product quality, finding the root cause can be challenging. Many of these variables maybe correlated, but for the human eye it is often very hard to distinguish correlation from causation. Advanced manufacturing intelligence tools distinguish random fluctuations from true effects such as root-causes or bottle necks in material flow thereby increasing yield and quality, while dramatically reducing the need for human intervention.
Enable New Views of Factory Processes
Processes, material flow, and complex manufacturing equipment are best understood by viewing from a variety of perspectives to unravel potential for optimization. To enable our clients to use data as an accelerator for yield increase and root cause analysis, we support a wide range of visualization and reporting solutions ranging from commercial tools such as Tableau, open-source solutions such as Grafana, Kibana or Shiny, or cloud analytics tools such as Azure Power BI. Where out-of-the-box solutions fall short, we also provide custom dashboard solutions serving individual business needs.
Our products enable seamless drilldown from the factory level down to specific tools or work items. This enables staff from operations, IT and process engineering to tackle complex data problems directly, efficiently and promptly as needed.
Benefits

An aspect of digital transformation in manufacturing

Enable administrators, operators and management to gain deep insights into your factory processes

Increase transparency using an interactive shop floor interface

Solve problems efficiently with anomaly detection & event classification

Monitor factory health in real-time with automatic data aggregation & KPI dashboards

Understand log events with enriched context information
What Manufacturers Say About Us
“Continuous optimization of production processes is a key factor for Vishay’s success in the field of electronic devices. To further improve these processes, Vishay works together with SYSTEMA in the area of automation and analytics.
SYSTEMA complements the deep expertise of Vishay’s manufacturing and engineering teams in an ideal way. Process quality improvements from the collaboration with SYSTEMA exceeded expectations. We thank SYSTEMA for their team spirit and support.“
Dr. Andreas Gondorf, Staff Engineer Advanced Process Control, Vishay Siliconix Itzehoe GmbH
SYSTEMA’s Approach
SYSTEMA goes beyond providing software licensing. We are solutions consultants who partner with and advise our clients as they pursue digital transformation strategies. We begin by gaining a comprehensive understanding of our client’s business. During on-site workshops, we work together with our clients to identify, gather, and prioritize requirements, formulate KPIs, and align the vision and objectives with stakeholders (management, production, IT, enterprise management, etc.). From there, we typically strive to first develop and provide a proof-of-concept as a basis for further iteration and development into a final solution for testing and future deployment. Our team has a broad global presence and we are prepared to support your initiatives, large and small, to efficiently realize the benefits of digital transformation in manufacturing.
Data-centric projects rely on a close – and often – agile feedback loop including all relevant players in the factory. SYSTEMA’s true strength is in coupling our extensive manufacturing IT experience with our ability to work closely with equipment operators, process engineers, operations, factory IT and management to understand the unique dynamics of each manufacturing environment and identify the most business-critical questions and use-cases that will drive success.
Our data teams work iteratively to address challenges and assess achievements as determined by client objectives and project requirements. SYSTEMA’s broad range of skill and experience with digital transformation in high tech manufacturing environments allows us to staff projects appropriately, whether data gathering through the MES, material tracking systems, tool or shop floor sensors, ERP or SPC systems. We develop our analytics products and solutions such that they can interface with your existing analytics stack. We also provide architecture guidance to build new manufacturing solutions from ground up using the latest technologies and accepted best practices.